About the State of AI Cybersecurity Report
Darktrace surveyed 1,800 CISOs, security leaders, administrators, and practitioners from industries around the globe. Our research was conducted to understand how the adoption of new AI-powered offensive and defensive cybersecurity technologies are being managed by organizations.
This blog continues the conversation from “The State of AI in Cybersecurity: Unveiling Global Insights from 1,800 Security Practitioners”. This blog will focus on security professionals’ understanding of AI technologies in cybersecurity tools.
To access download the full report, click here.
How familiar are security professionals with supervised machine learning
Just 31% of security professionals report that they are “very familiar” with supervised machine learning.
Many participants admitted unfamiliarity with various AI types. Less than one-third felt "very familiar" with the technologies surveyed: only 31% with supervised machine learning and 28% with natural language processing (NLP).
Most participants were "somewhat" familiar, ranging from 46% for supervised machine learning to 36% for generative adversarial networks (GANs). Executives and those in larger organizations reported the highest familiarity.
Combining "very" and "somewhat" familiar responses, 77% had familiarity with supervised machine learning, 74% generative AI, and 73% NLP. With generative AI getting so much media attention, and NLP being the broader area of AI that encompasses generative AI, these results may indicate that stakeholders are understanding the topic on the basis of buzz, not hands-on work with the technologies.
If defenders hope to get ahead of attackers, they will need to go beyond supervised learning algorithms trained on known attack patterns and generative AI. Instead, they’ll need to adopt a comprehensive toolkit comprised of multiple, varied AI approaches—including unsupervised algorithms that continuously learn from an organization’s specific data rather than relying on big data generalizations.
Different types of AI
Different types of AI have different strengths and use cases in cyber security. It’s important to choose the right technique for what you’re trying to achieve.
Supervised machine learning: Applied more often than any other type of AI in cyber security. Trained on human attack patterns and historical threat intelligence.
Large language models (LLMs): Applies deep learning models trained on extremely large data sets to understand, summarize, and generate new content. Used in generative AI tools.
Natural language processing (NLP): Applies computational techniques to process and understand human language.
Unsupervised machine learning: Continuously learns from raw, unstructured data to identify deviations that represent true anomalies.
What impact will generative AI have on the cybersecurity field?
More than half of security professionals (57%) believe that generative AI will have a bigger impact on their field over the next few years than other types of AI.
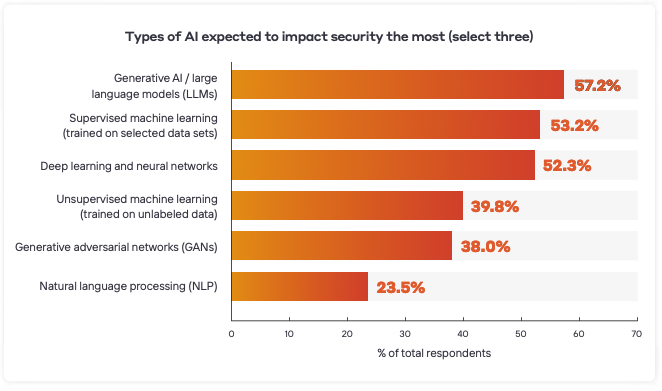
Security stakeholders are highly aware of generative AI and LLMs, viewing them as pivotal to the field's future. Generative AI excels at abstracting information, automating tasks, and facilitating human-computer interaction. However, LLMs can "hallucinate" due to training data errors and are vulnerable to prompt injection attacks. Despite improvements in securing LLMs, the best cyber defenses use a mix of AI types for enhanced accuracy and capability.
AI education is crucial as industry expectations for generative AI grow. Leaders and practitioners need to understand where and how to use AI while managing risks. As they learn more, there will be a shift from generative AI to broader AI applications.
Do security professionals fully understand the different types of AI in security products?
Only 26% of security professionals report a full understanding of the different types of AI in use within security products.
Confusion is prevalent in today’s marketplace. Our survey found that only 26% of respondents fully understand the AI types in their security stack, while 31% are unsure or confused by vendor claims. Nearly 65% believe generative AI is mainly used in cybersecurity, though it’s only useful for identifying phishing emails. This highlights a gap between user expectations and vendor delivery, with too much focus on generative AI.
Key findings include:
- Executives and managers report higher understanding than practitioners.
- Larger organizations have better understanding due to greater specialization.
As AI evolves, vendors are rapidly introducing new solutions faster than practitioners can learn to use them. There's a strong need for greater vendor transparency and more education for users to maximize the technology's value.
To help ease confusion around AI technologies in cybersecurity, Darktrace has released the CISO’s Guide to Cyber AI. A comprehensive white paper that categorizes the different applications of AI in cybersecurity. Download the White Paper here.
Do security professionals believe generative AI alone is enough to stop zero-day threats?
No! 86% of survey participants believe generative AI alone is NOT enough to stop zero-day threats
This consensus spans all geographies, organization sizes, and roles, though executives are slightly less likely to agree. Asia-Pacific participants agree more, while U.S. participants agree less.
Despite expecting generative AI to have the most impact, respondents recognize its limited security use cases and its need to work alongside other AI types. This highlights the necessity for vendor transparency and varied AI approaches for effective security across threat prevention, detection, and response.
Stakeholders must understand how AI solutions work to ensure they offer advanced, rather than outdated, threat detection methods. The survey shows awareness that old methods are insufficient.