What is Living off the Land attack?
In the face of increasingly vigilant security teams and adept defense tools, attackers are continually looking for new ways to circumvent network security and gain access to their target environments. One common tactic is the leveraging of readily available utilities and services within a target organization’s environment in order to move through the kill chain; a popular method known as living off the land (LotL). Rather than having to leverage known malicious tools or write their own malware, attackers are able to easily exploit the existing infrastructure of their targets.
The Medusa ransomware group in particular are known to extensively employ LotL tactics, techniques and procedures (TTPs) in their attacks, as one Darktrace customer in the US discovered in early 2024.
What is Medusa Ransomware?
Medusa ransomware (not to be confused with MedusaLocker) was first observed in the wild towards the end of 2022 and has been a popular ransomware strain amongst threat actors since 2023 [1]. Medusa functions as a Ransomware-as-a-Service (RaaS) platform, providing would-be attackers, also know as affiliates, with malicious software and infrastructure required to carry out disruptive ransomware attacks. The ransomware is known to target organizations across many different industries and countries around the world, including healthcare, education, manufacturing and retail, with a particular focus on the US [2].
How does Medusa Ransomware work?
Medusa affiliates are known to employ a number of TTPs to propagate their malware, most prodominantly gaining initial access by exploiting vulnerable internet-facing assets and targeting valid local and domain accounts that are used for system administration.
The ransomware is typically delivered via phishing and spear phishing campaigns containing malicious attachments [3] [4], but it has also been observed using initial access brokers to access target networks [5]. In terms of the LotL strategies employed in Medusa compromises, affiliates are often observed leveraging legitimate services like the ConnectWise remote monitoring and management (RMM) software and PDQ Deploy, in order to evade the detection of security teams who may be unable to distinguish the activity from normal or expected network traffic [2].
According to researchers, Medusa has a public Telegram channel that is used by threat actors to post any data that may have been stolen, likely in an attempt to extort organizations and demand payment [2].
Darktrace’s Coverage of Medusa Ransomware
Established Foothold and C2 activity
In March 2024, Darktrace /NETWORK identified over 80 devices, including an internet facing domain controller, on a customer network performing an unusual number of activities that were indicative of an emerging ransomware attack. The suspicious behavior started when devices were observed making HTTP connections to the two unusual endpoints, “wizarr.manate[.]ch” and “go-sw6-02.adventos[.]de”, with the PowerShell and JWrapperDownloader user agents.
Darktrace’s Cyber AI Analyst™ launched an autonomous investigation into the connections and was able to connect the seemingly separate events into one wider incident spanning multiple different devices. This allowed the customer to visualize the activity in chronological order and gain a better understanding of the scope of the attack.
At this point, given the nature and rarity of the observed activity, Darktrace /NETWORK's autonomous response would have been expected to take autonomous action against affected devices, blocking them from making external connections to suspicious locations. However, autonomous response was not configured to take autonomous action at the time of the attack, meaning any mitigative actions had to be manually approved by the customer’s security team.
Internal Reconnaissance
Following these extensive HTTP connections, between March 1 and 7, Darktrace detected two devices making internal connection attempts to other devices, suggesting network scanning activity. Furthermore, Darktrace identified one of the devices making a connection with the URI “/nice ports, /Trinity.txt.bak”, indicating the use of the Nmap vulnerability scanning tool. While Nmap is primarily used legitimately by security teams to perform security audits and discover vulnerabilities that require addressing, it can also be leveraged by attackers who seek to exploit this information.

Darktrace observed actors using multiple credentials, including “svc-ndscans”, which was also seen alongside DCE-RPC activity that took place on March 1. Affected devices were also observed making ExecQuery and ExecMethod requests for IWbemServices. ExecQuery is commonly utilized to execute WMI Query Language (WQL) queries that allow the retrieval of information from WI, including system information or hardware details, while ExecMethod can be used by attackers to gather detailed information about a targeted system and its running processes, as well as a tool for lateral movement.
Lateral Movement
A few hours after the first observed scanning activity on March 1, Darktrace identified a chain of administrative connections between multiple devices, including the aforementioned internet-facing server.
Cyber AI Analyst was able to connect these administrative connections and separate them into three distinct ‘hops’, i.e. the number of administrative connections made from device A to device B, including any devices leveraged in between. The AI Analyst investigation was also able to link the previously detailed scanning activity to these administrative connections, identifying that the same device was involved in both cases.

On March 7, the internet exposed server was observed transferring suspicious files over SMB to multiple internal devices. This activity was identified as unusual by Darktrace compared to the device's normal SMB activity, with an unusual number of executable (.exe) and srvsvc files transferred targeting the ADMIN$ and IPC$ shares.

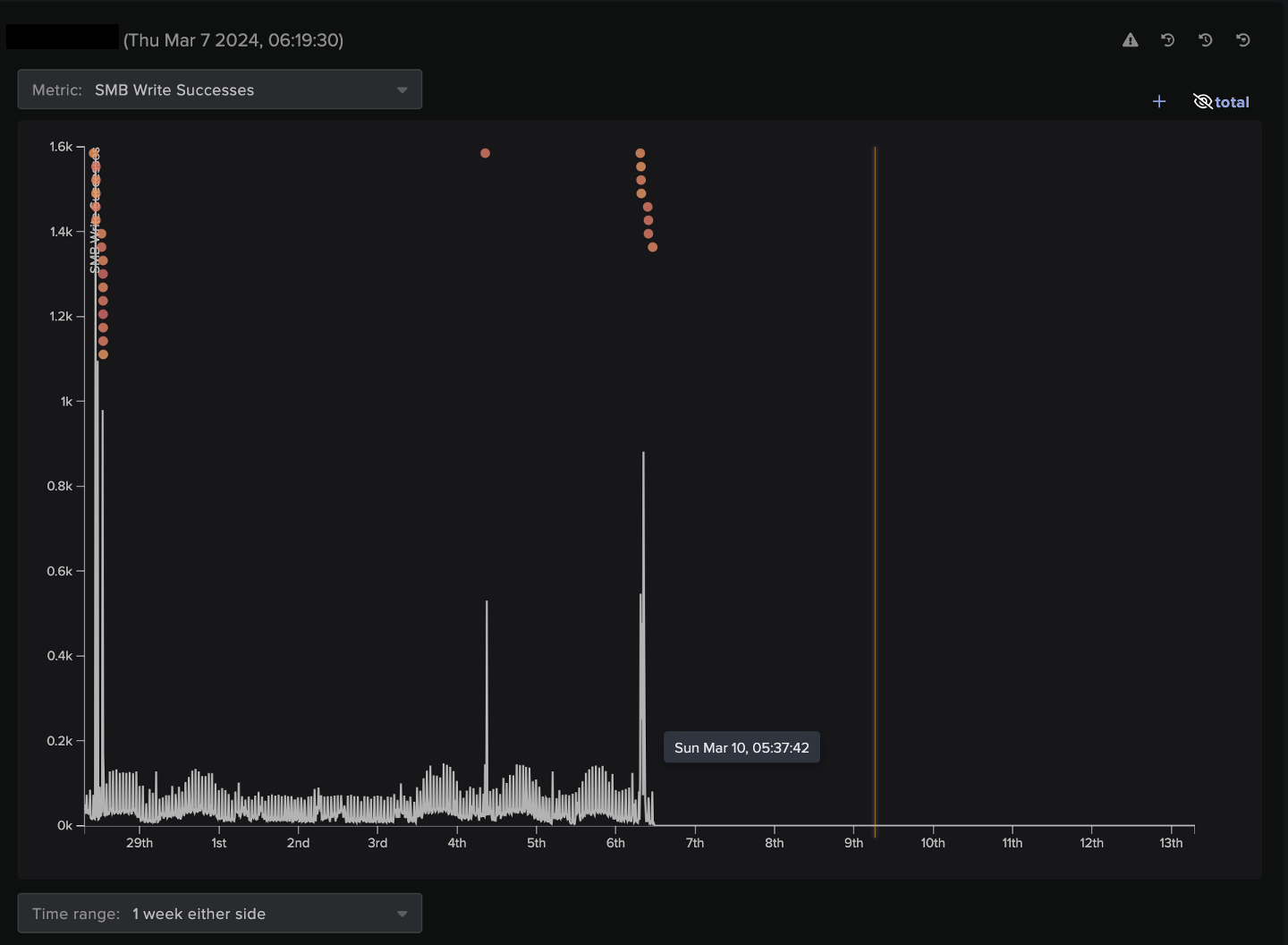
The threat actor was also seen writing SQLite3*.dll files over SMB using a another credential this time. These files likely contained the malicious payload that resulted in the customer’s files being encrypted with the extension “.s3db”.

Encryption of Files
Finally, Darktrace observed the malicious actor beginning to encrypt and delete files on the customer’s environment. More specifically, the actor was observed using credentials previously seen on the network to encrypt files with the aforementioned “.s3db” extension.

After that, Darktrace observed the attacker encrypting files and appending them with the extension “.MEDUSA” while also dropping a ransom note with the file name “!!!Read_me_Medusa!!!.txt”


At the same time as these events, Darktrace observed the attacker utilizing a number of LotL techniques including SSL connections to “services.pdq[.]tools”, “teamviewer[.]com” and “anydesk[.]com”. While the use of these legitimate services may have bypassed traditional security tools, Darktrace’s anomaly-based approach enabled it to detect the activity and distinguish it from ‘normal’ network activity. It is highly likely that these SSL connections represented the attacker attempting to exfiltrate sensitive data from the customer’s network, with a view to using it to extort the customer.
![Cyber AI Analyst’s detection of “services.pdq[.]tools” usage.](https://cdn.prod.website-files.com/626ff4d25aca2edf4325ff97/66ec6eeedc6c2eb04b59b9aa_66675ec67a26a321c19aa6e1_Screenshot%25202024-06-10%2520at%25201.14.55%2520PM.png)
If this customer had been subscribed to Darktrace's Proactive Threat Notification (PTN) service at the time of the attack, they would have been promptly notified of these suspicious activities by the Darktrace Security Operation Center (SOC). In this way they could have been aware of the suspicious activities taking place in their infrastructure before the escalation of the compromise. Despite this, they were able to receive assistance through the Ask the Expert service (ATE) whereby Darktrace’s expert analyst team was on hand to assist the customer by triaging and investigating the incident further, ensuring the customer was well equipped to remediate.
As Darktrace /NETWORK's autonomous response was not enabled in autonomous response mode, this ransomware attack was able to progress to the point of encryption and data exfiltration. Had autonomous response been properly configured to take autonomous action, Darktrace would have blocked all connections by affected devices to both internal and external endpoints, as well as enforcing a previously established “pattern of life” on the device to stop it from deviating from its expected behavior.
Conclusion
The threat actors in this Medusa ransomware attack attempted to utilize LotL techniques in order to bypass human security teams and traditional security tools. By exploiting trusted systems and tools, like Nmap and PDQ Deploy, attackers are able to carry out malicious activity under the guise of legitimate network traffic.
Darktrace’s Self-Learning AI, however, allows it to recognize the subtle deviations in a device’s behavior that tend to be indicative of compromise, regardless of whether it appears legitimate or benign on the surface.
Further to the detection of the individual events that made up this ransomware attack, Darktrace’s Cyber AI Analyst was able to correlate the activity and collate it under one wider incident. This allowed the customer to track the compromise and its attack phases from start to finish, ensuring they could obtain a holistic view of their digital environment and remediate effectively.
Credit to Maria Geronikolou, Cyber Analyst, Ryan Traill, Threat Content Lead
Appendices
Darktrace DETECT Model Detections
Anomalous Connection / SMB Enumeration
Device / Anomalous SMB Followed By Multiple Model Alerts
Device / Suspicious SMB Scanning Activity
Device / Attack and Recon Tools
Device / Suspicious File Writes to Multiple Hidden SMB Share
Compromise / Ransomware / Ransom or Offensive Words Written to SMB
Device / Internet Facing Device with High Priority Alert
Device / Network Scan
Anomalous Connection / Powershell to Rare External
Device / New PowerShell User Agent
Possible HTTP Command and Control
Extensive Suspicious DCE-RPC Activity
Possible SSL Command and Control to Multiple Endpoints
Suspicious Remote WMI Activity
Scanning of Multiple Devices
Possible Ransom Note Accessed over SMB
List of Indicators of Compromise (IoCs)
IoC – Type – Description + Confidence
207.188.6[.]17 - IP address - C2 Endpoint
172.64.154[.]227 - IP address - C2 Endpoint
wizarr.manate[.]ch - Hostname - C2 Endpoint
go-sw6-02.adventos[.]de. Hostname - C2 Endpoint
.MEDUSA - File extension - Extension to encrypted files
.s3db - File extension - Created file extension
SQLite3-64.dll - File - Used tool
!!!Read_me_Medusa!!!.txt - File - Ransom note
Svc-ndscans - Credential - Possible compromised credential
Svc-NinjaRMM - Credential - Possible compromised credential
MITRE ATT&CK Mapping
Discovery - File and Directory Discovery - T1083
Reconnaissance - Scanning IP - T1595.001
Reconnaissance - Vulnerability Scanning - T1595.002
Lateral Movement -Exploitation of Remote Service - T1210
Lateral Movement - Exploitation of Remote Service - T1210
Lateral Movement - SMB/Windows Admin Shares - T1021.002
Lateral Movement - Taint Shared Content - T1080
Execution - PowerShell - T1059.001
Execution - Service Execution - T1059.002
Impact - Data Encrypted for Impact - T1486